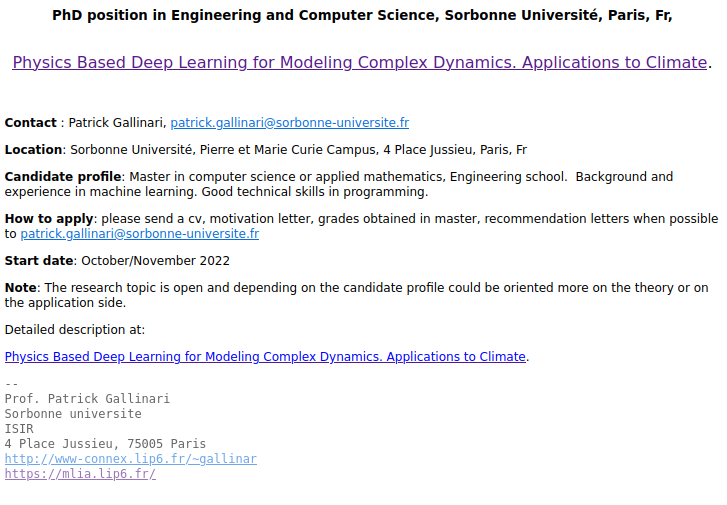
Université de Lorraine
Type d’emploi : poste d’ingénieur en informatique (H/F 2 ans à temps plein)
Lieu : Nancy, LORIA, équipe Orpailleur (contact : amedeo.napoli@loria.fr)
Niveau : Ingénieur en informatique (bac+5, Master ou école d’ingénieur)
(** English below **)
Missions et activités.
Dans le cadre du projet Kesaio, un poste d’ingénieur est à pourvoir pour une durée de 2 ans dans l’équipe Orpailleur au LORIA Nancy.
Le projet Kesaio a pour objectif de définir et d’implémenter une méthode semi-automatique de conception et d’enrichissement d’ontologies à partir de documents accessibles sur le web des données.
Dans ce projet, l’ingénierie des connaissances tient une place majeure et fait appel à des techniques de découverte de connaissances (recherche de définitions de concepts dans des données RDF), de représentation des connaissances et de raisonnement. Ainsi, il est envisagé d’utiliser des méthodes de fouille de motifs et l’analyse formelle de concepts (FCA). De plus, des méthodes de conception et de gestion de graphes de connaissances seront également mises en oeuvre.
Contexte de travail
Le travail se fera au Laboratoire LORIA, dans l’équipe Orpailleur (https://orpailleur.loria.fr/), qui possède un savoir-faire et une expertise en découverte de connaissances, en fouille de données et apprentissage, représentation des connaissances et raisonnement, et en conception semi-automatique d’ontologies.
Dans ce projet, l’équipe Orpailleur collabore avec l’entreprise Airudit, maître d’oeuvre du projet Kesaio, et qui développe entre autres des assistants conversationnels (voir https://www.airudit.com/fr-FR/).
Candidature et compétences.
Le candidat doit avoir un Master en informatique ou un diplôme d’ingénieur (bac+5) avec une composante forte en informatique et/ou mathématiques appliquées.
Des connaissances en découverte de connaissances, en apprentissage, en représentation des connaissances, en formalisation du raisonnement et en ingénierie des ontologies seront fortement appréciées.
La candidature doit impérativement se faire sur le Portail Emploi du CNRS (https://emploi.cnrs.fr/) et doit comprendre un curriculum vitae récent, une lettre de motivation, deux lettres de recommandation ou deux noms de référents, ainsi qu’un relevé des notes des trois dernières années d’étude (L3, M1 et M2 ou école d’ingénieurs).
Contact : Amedeo Napoli (amedeo.napoli@loria.fr)
BiOceanOr
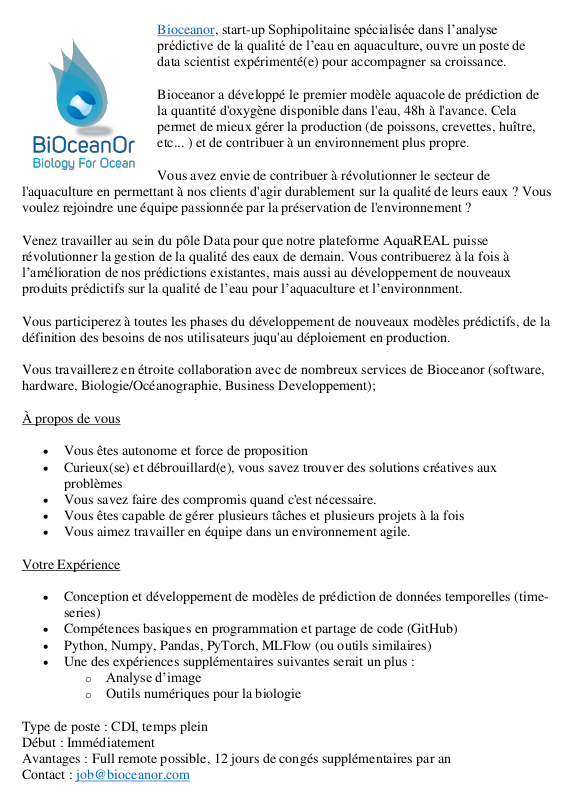
INRIA
Safran Tech / École Polytechnique
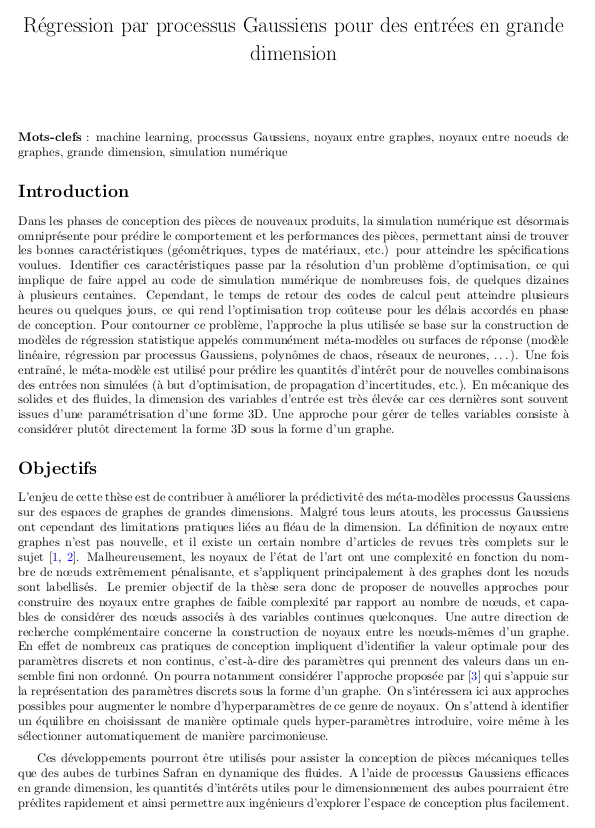
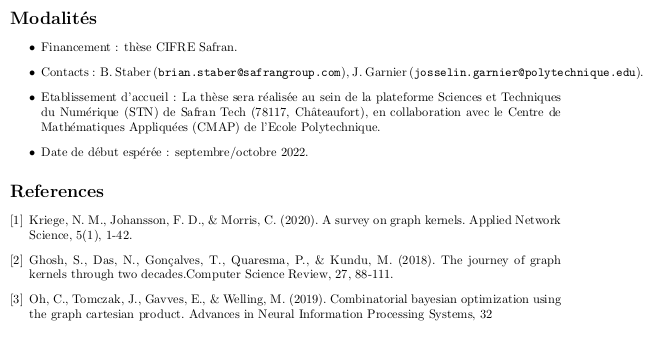
Orange Labs
Offre de thèse aux laboratoires Orange Labs et IRISA (à Lannion) : Deep learning pour l’estimation de l’impact des drones sur un réseau mobile
Résumé
L’objectif de cette thèse est de modéliser et d’évaluer l’impact de trajectoires de drones connectés sur un réseau d’accès radio. Ceci doit se faire en tenant compte des axes routiers et des couvertures cellulaires d’un ou de plusieurs opérateurs. Le but du modèle sera de prendre en compte des trajets prévus de drones pour (1) minimiser l’impact en termes de trafic sur un RAN (radio access network) donné, (2) éviter les interférences entre drones et zones sensibles, et (3) conserver une bonne QoE pour les équipements de l’utilisateur (UE).
Compétences requises : connaissance des réseaux mobiles, Deep Learning (idéalement PyTorch ou Tensorflow), théorie des graphes, Python, Shell, Matlab serait un plus, bonne communication en anglais à l’oral et à l’écrit, travail en équipe, curiosité et ouverture d’esprit.
Comment candidater ?
https://orange.jobs/jobs/offer.do?joid=112418&lang=FR
Plus de détails :
En Français : détails
En Anglais: détails
INRIA
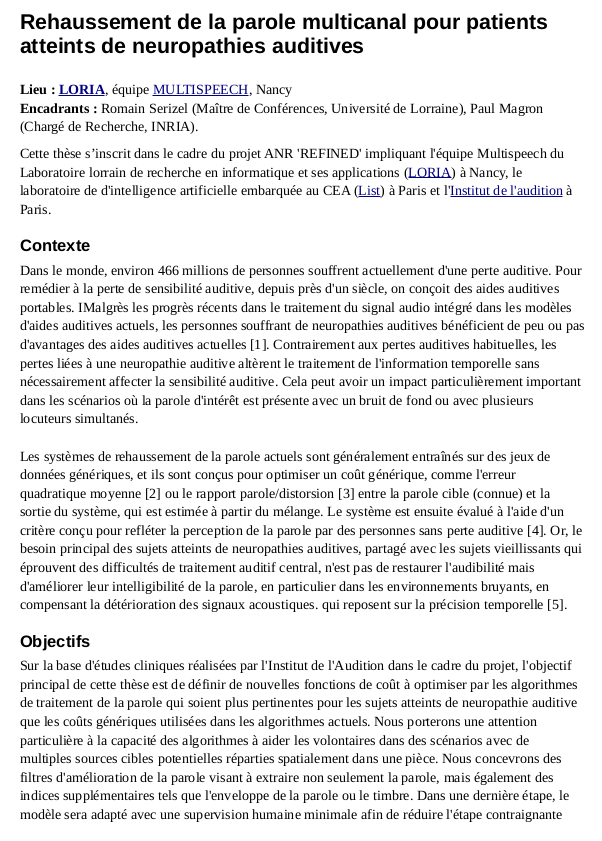
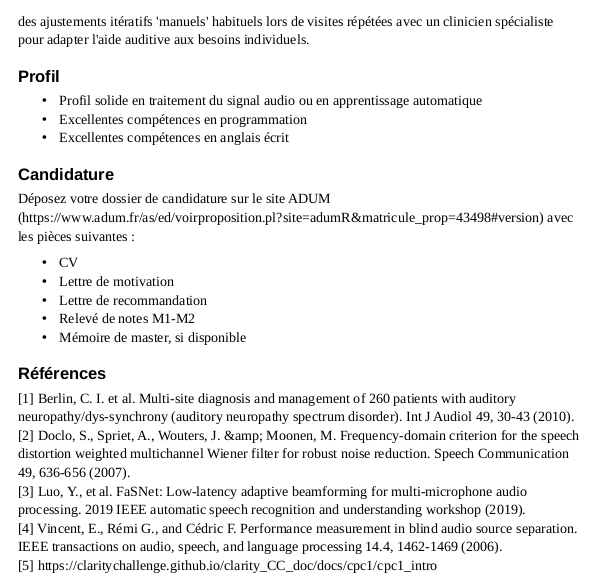
Université de Franche-Comté
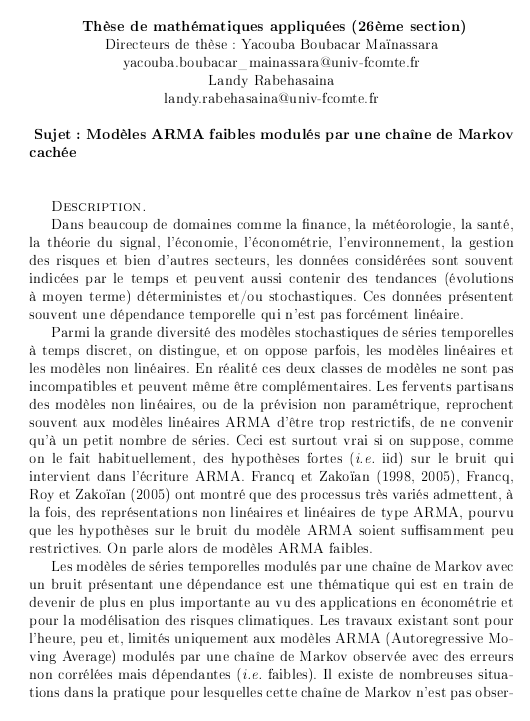
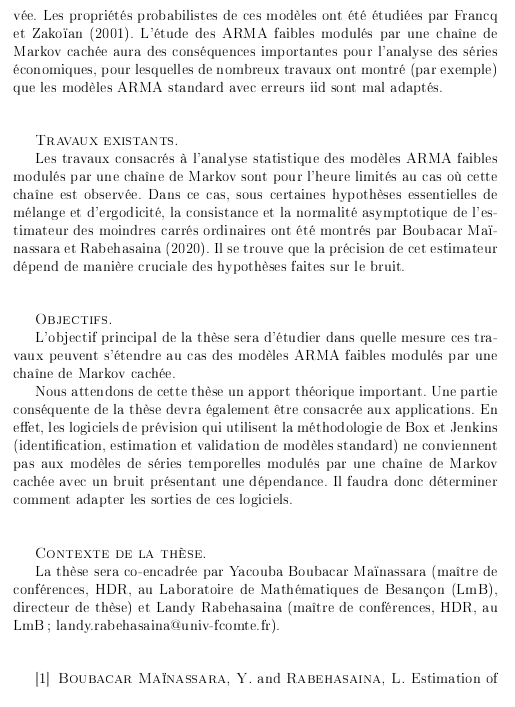
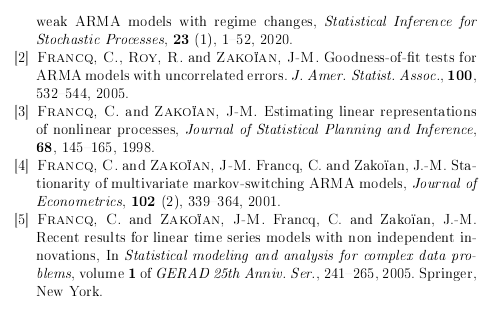
Université d’Artois
A Ph.D. position in Artificial Intelligence and Machine Learning is open at the CRIL lab. This Ph.D. fellowship shall be funded for 3 years from October 2022.
Title: Learning Interpretable Circuits
Overview: In recent years, there has been a growing interest in the design of statistical learning algorithms for interpretable models, which are not only accurate but also understandable by human users. A related and desirable property is explainability, which refers to the computational ability of predictive models to explain their predictions in intelligible terms.
In this setting, decision trees are of paramount importance, as they can be easily read by recursively breaking a choice into sub-choices until a decision is reached. Although the problem of learning decision trees is NP-hard, several optimization approaches have been proposed for identifying a decision tree that minimizes some regularized risk objective (e.g. [1,2,3]).
Beyond decision trees, various classes of (boolean or arithmetic) circuits are also endowed with interpretability and explainability properties. These models are indeed able to efficiently handle a wide variety of explanation queries, by exploiting the decomposability property on the logical “and’’ gates, and the determinism of “or’’ gates [4]. Well-known classes of interpretable circuits include ordered decision diagrams, affine decision trees, and decomposable negation normal form representations.
Although the problem of learning circuits has been studied in the literature (e.g. [5,6]), very little is known about learning interpretable circuits. The focus of this thesis proposal is to address this issue, by exploring several directions of research. One of them is to identify the learnability of several subclasses of interpretable circuits. Another important perspective is to exploit constraint-based or MILP solvers for extracting optimal – or quasi-optimal – interpretable circuits. Finally, since decision circuits can easily be extended to semi-ring networks, a final topic is to extend results about binary classification to other learning tasks, such as regression or density estimation.
References:
[1] Nina Narodytska, Alexey Ignatiev, Filipe Pereira, João Marques-Silva: Learning Optimal Decision Trees with SAT. IJCAI 2018: 1362-1368.
[2] Emir Demirovic, Anna Lukina, Emmanuel Hebrard, Jeffrey Chan, James Bailey, Christopher Leckie, Kotagiri Ramamohanarao, Peter J. Stuckey: MurTree: Optimal Decision Trees via Dynamic Programming and Search. J. Mach. Learn. Res. 23: 26:1-26:47 (2022).
[3] Valentina Zantedeschi, Matt J. Kusner, Vlad Niculae: Learning Binary Decision Trees by Argmin Differentiation. ICML 2021: 12298-12309.
[4] Gilles Audemard, Frédéric Koriche, Pierre Marquis: On Tractable XAI Queries based on Compiled Representations. KR 2020: 838-849.
|5] Nathan Linial, Yishay Mansour, Noam Nisan. Constant depth circuits, Fourier transform, and learnability. FOCS 1989: 574–579.
[6] Eran Malach, Shai Shalev-Shwartz. Learning Boolean Circuits with Neural Networks. CoRR abs/1910.11923 (2019).
Profile: The candidate should have a Master or similar degree in computer science. As the thesis proposal lies at the intersection of explainable artificial intelligence, combinatorial optimization, and machine learning, the candidate should have a strong background in some of these topics.
Contacts: Frederic Koriche (koriche@cril.fr), Jean-Marie Lagniez (lagniez@cril.fr) and Stefan Mengel (mengel@cril.fr).
Télécom Paris
Dear all,
We have multiple openings for PhD students, Postdoctoral Researchers and R&D Engineers at Télécom Paris, Institut polytechnique de Paris, in the “Signal, Statistics and Learning (S2A) team.
All positions are located at Telecom Paris, 19 place Marguerite Perey, 91120 Palaiseau, France.
Start of the positions: October/November 2022 (for PhDs/Engineer), January 2023 for PostDoc
Subject:
The positions will be a part of the ERC Advanced (2022) – HI-Audio (Hybrid and Interpretable Deep neural audio machines)project, which aims at building hybrid deep approaches combining parameter-efficient and interpretable models with modern resource-efficient deep neural architectures with applications in speech/audio scene analysis, music information retrieval and sound transformation and synthesis.
The potential topics include (and are not limited to):
– Deep generative models, adversarial learning
– Attention-based models and curriculum learning
– Statistical/deterministic audio models (signal models, sound propagation models,…)
– Music Information Retrieval software platform development (R&D Engineer position)
Candidate Profile:
– For the Phd positions: A masters degree in applied mathematics, datascience/computer scienceor speech/audio/music processing is required.
– For the Postdoc position: PhD degree and publications in theory or applications of machine learning, generative modelling, discrete optimal transport or signal processing, ideally with applications to Speech/Audio/Music signals.
– Master internship positions will also be open in early 2023.
Télécom Paris, and the S2A team:
The S2A team gathers 18 permanent faculties covering a wide variety of research topics including Statistics, Probabilistic modeling, Machine learning, Data science, Audio and social signal processing. On the overall, Télécom Paris’ research counts 19 research teams and covers various domains in computer science and networks, applied mathematics, electronics, image, data, signals and economic and social sciences. Télécom Paris (https://www.telecom-paris.fr/en/home) is a member of IMT (Institut Mines-Télécom), and is a founding member of the Institut Polytechnique de Paris (IP Paris, https://www.ip-paris.fr/en), a world-class scientific and technological institution which is a partnership between five prestigious French engineering schools with HEC as a key partner.
Application:
– There is no specific deadline. Applications are welcome until all positions are filled.
– In the application, please send a resume, a motivation letter (and full transcript grades for Phd/Engineer positions) to Gaël Richard, firstname.lastname@telecom-paris.fr. At least one reference letter will be asked in a second step.