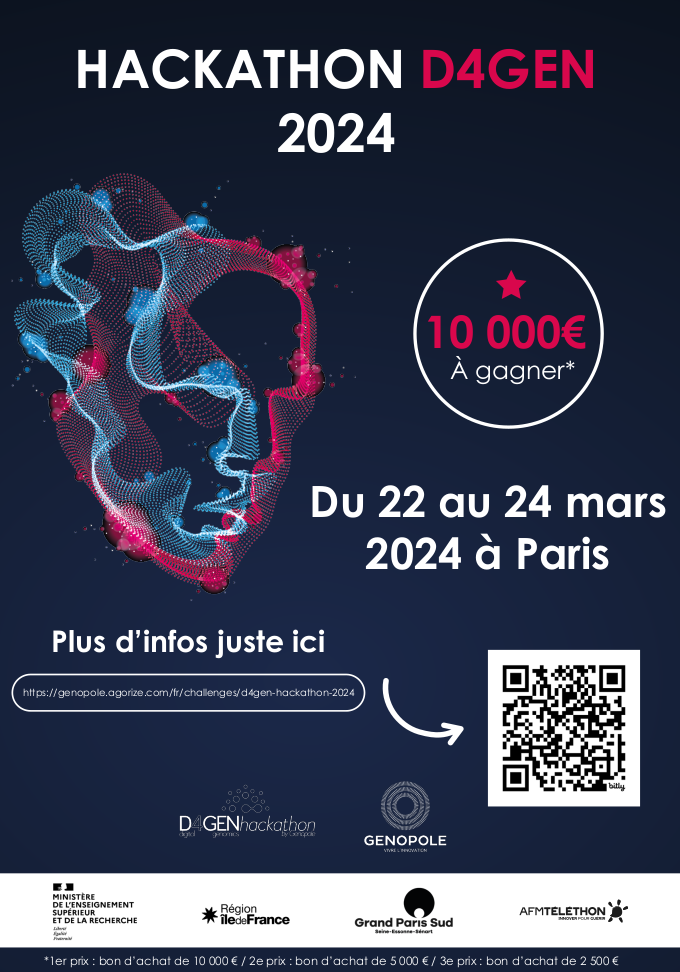
Hackaton IA générative ACPR
IT4GREEN
Genhack 2022 : Hackathon for Generative Modelling
Geomstats Hackathon
Hackathon Intelligence Artificielle du 13 au 15 mai
AI-Cup
ICLR 2022 Challenge of Differential Geometry and Learning
We are co-organizing a computational challenge at the ICLR 2022 workshop of geometry and topology. The goal is to push forward the field of computational differential geometry by asking participants to contribute statistics and learning algorithms on manifolds. The guidelines of the challenge can be found on its GitHub repository. The deadline is April 4th, 2022. Would you know people who could be interested in participating in the challenge? It can be a good opportunity to jump into coding geometry while being rewarded for it: - $3500 in prizes, - public recognition with an award at ICLR 2022, and - co-authorship on the paper summarizing the findings of the challenge. Looking forward to your submissions!
ICLR Workshop on Geometrical and Topological Representation Learning
Hi! PARIS Hackathon on “AI and sustainability”
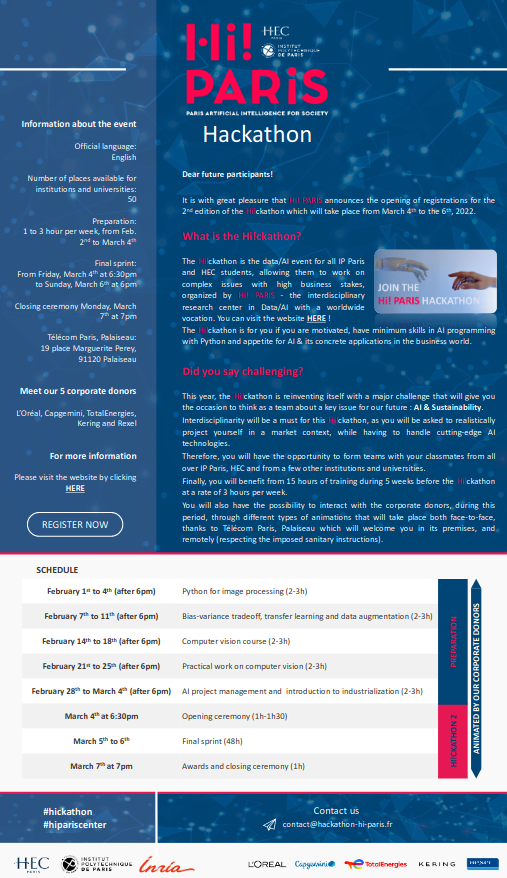
Hi! PARIS Hackathon on “AI and sustainability”
- Competition on March 4-6, 2022
- Skill building and interaction with Hi! PARIS corporate donors from Feb 1-Mar 4, 2022
Website: https://hackathon-hi-paris.fr/
Registration to the Hi! PARIS Hackathon is open until February 14, 2022. This year, the event is also widely open to students of university courses. Outside HEC and IP PARIS.
The Hi!ckathon will give you the possibility to work on an AI problem that is both complex and has high business and societal stakes, in line with the AI & Sustainability theme. The official language of the event is English.
You are motivated, you feel to have a minimum of skills in AI programming with Python, you are interested in AI and its concrete applications in the business world, you would like to work in mixed teams with other students from IP Paris, HEC and other universities à Register now!
The Hi! PARIS hackathon, the format
- a Hackathon weekend from March 5 to 6, 2022 à at Telecom Paris, Palaiseau
- 5 weeks of skill building through testimonials from Hi! PARIS Corporate donors (L’Oréal, Capgemini, TotalEnergies, Kering and Rexel), exercises and Q&A from February 1 to March 4, 2022, weekly investment ~2h à remotely
Registration is simple, you just have to fill in the dedicated form available on the HFactory platform. You will receive a validation e-mail after your profile has been examined.
Registration link, leaflet and updated program on https://hackathon-hi-paris.fr/