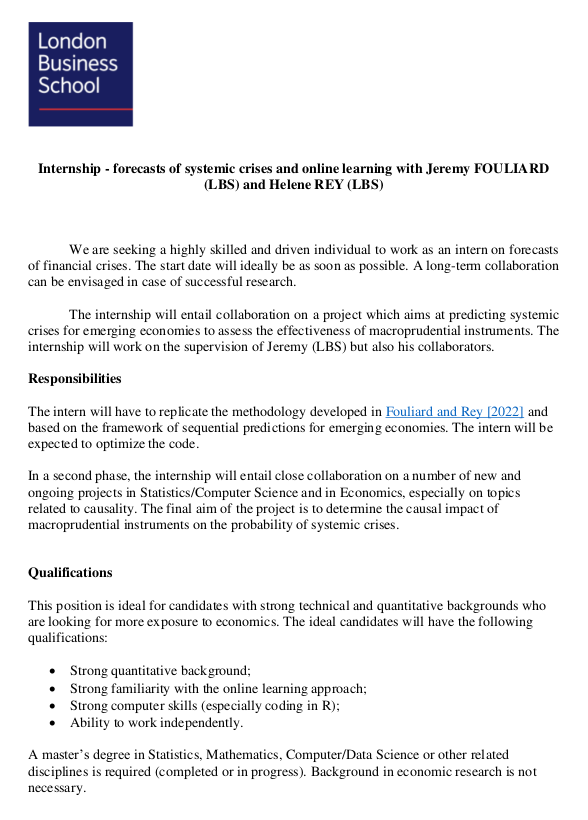
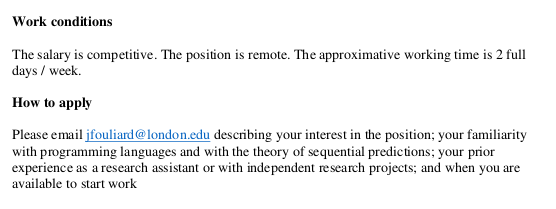
M2 Apprentissage et Algorithmes (M2A)
Master Mathématiques et Applications / Master Informatique – Sorbonne Université
Dear all,
Apologies for cross-posting.
We have multiple openings for Interns, PhD students, and Postdoctoral Researchers at the SIERRA Machine Learning Team (Paris, France) which is a joint laboratory between INRIA, the CS Dept. at Ecole Normale Superieure, and CNRS.
Subject:All the positions will be a part of the ERC Starting Grant (2021) – DYNASTY (Dynamics-Aware Theory of Deep Learning) project, which focuses on various aspects of Deep Learning Theory. The potential topics include (and are not limited to):- Generalization bounds for neural networks- Implicit regularization- Non-convex optimization- Network compression
Candidate Profile:
– For the Internship positions: Enrollment at a masters/PhD program is required, preferably pure/applied mathematics or statistics. – For the Phd positions: A masters degree in applied mathematics, statistics, or computer science is required. (A direct PhD after undergrad is not possible).- For the Postdoc positions: PhD degree (and publications) in ML Theory or related fields such as applied mathematics (preferably applied probability, stochastic analysis, operations research), statistics, computer science.
A background on dynamical systems / fractal geometry / heavy-tailed stochastic recursions will be a plus.
I will not consider candidates that only have an applications background.
The SIERRA Team:
The researcher will be hosted at the INRIA Paris center, in downtown Paris.
A non-exhaustive list of topics that are currently investigated by the researchers of the SIERRA team includes:
– Large scale non-smooth optimization methods.
– Distributed optimization methods (asynchronous stochastic gradient optimization).
– First order-methods.
– Nonconvex optimization.- Kernel methods.
Application Process / Deadline:
– There is no specific deadline. The applications will be considered on a “first-come-first-serve” basis. – Internships typically take 3-6 months. The timing can be flexible. – In the application, please send a resume, a motivation letter, and at least one reference letter to Umut Simsekli — “umut.simsekli at inria.fr“.
Best regards,Umut Simsekli–https://www.di.ens.fr/~simsekli/
Title: Evolutional Deep Neural Network (EDNN) for Resolution of High-Dimensional Partial Differential Equations
Summary: Machine-learning (ML) holds significant promise in revolutionizing a wide range of applications, in particular in the domain of multi-scale and multi-physics problems. Success in realizing the promise of ML is predicated on the availability of training data, which are often obtained from scientific computations. Conventional approaches to solving the equations of physics require difficult and specialized software development, grid generation and adaptation, and the use of specialized data and software pipelines that differ from those adopted in ML. A disruptive new approach is Evolutional Deep Neural Networks (EDNN, pronounced “Eden”) which leverages the software and hardware infrastructure used in ML to replace conventional computational methods, and to tackle their shortcomings. EDNN is unique because it does not rely on training to express known solutions, but rather the network parameters evolve using the governing physical laws such that the network can predict the evolution of the physical system. In the proposed effort, we will develop the EDNN framework to solve high-dimensional partial differential equations, used to model a vast range of phenomena in economics, finance, operational research, and multi-phase fluid dynamics, where population balance equations govern phenomena as diverse as aerosol transmission of airborne pathogens or mixing enhancement in energy conversion devices.
Contact: Taraneh SAYADI <taraneh.sayadi@sorbonne-universite.fr