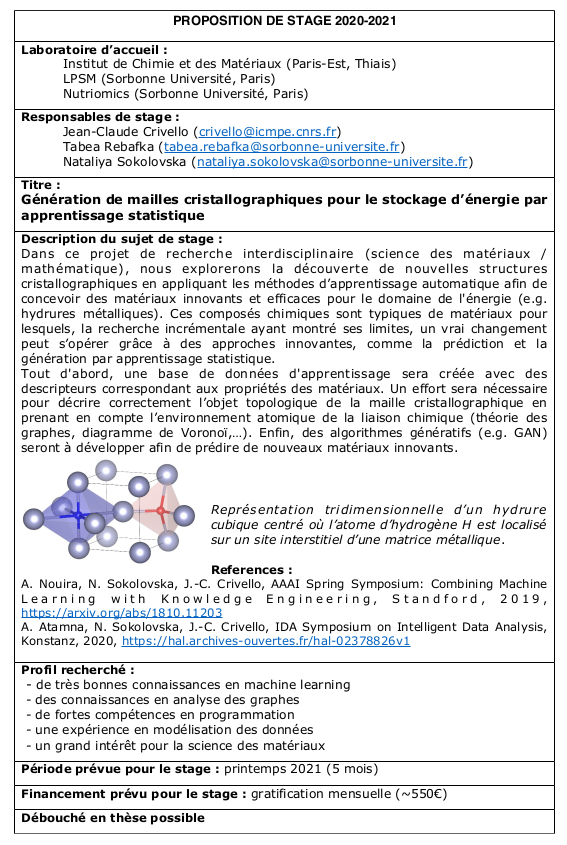
Sorbonne Université
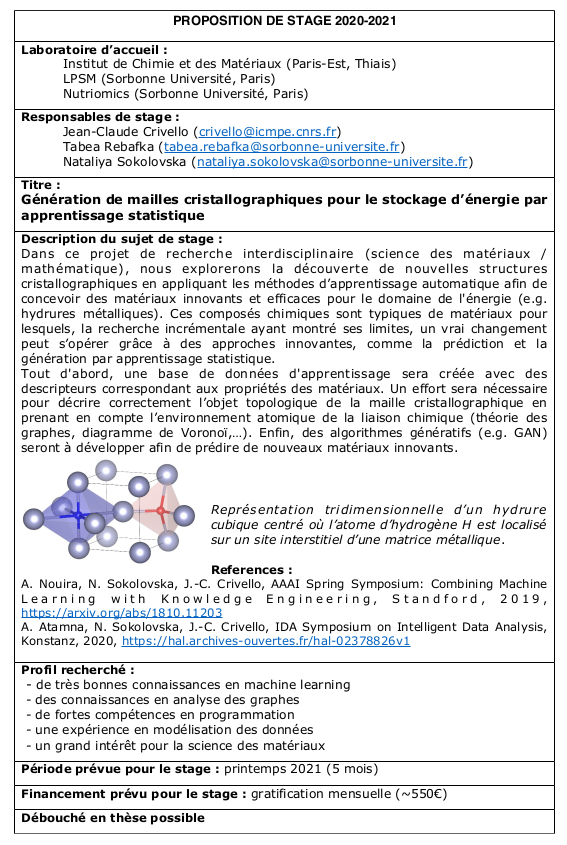
M2 Apprentissage et Algorithmes (M2A)
Master Mathématiques et Applications / Master Informatique – Sorbonne Université
Voir l’archive.
The thesis will take place in the tri-lateral (French-German-Japanese) RECOMP research action that aims at ensuring the artificial intelligent agents compliance with legal and ethical norms. More specifically, the proposed research is dedicated to computational ethics i.e. to model ethical concepts and ethical reasoning with AI tools in the context of the RECOMP project. The practical goal will be to design real time ethical supervisors for artificial agents, which are pieces of software that have to govern artificial agents, e.g. autonomous vehicles, robots or healthcare artificial agents, in order to ensure that they don’t infringe moral rules, except in specific situations where ethical norms are conflicting. This doesn’t mean that the agents that would result be, properly speaking, ethical, which would be ridiculously anthropocentric, but that such an ethical supervisor makes the agents’ behaviors to conform as far as possible to moral rules of conducts. The candidate will have to ensure the compatibility of the representation and the mechanism he will use with the representations used by the other partners of the project, which are German and Japanese. The work will be done in ACASA-LIP6 team, on the Pierre and Marie Curie Campus, under the supervision of Jean-Gabriel Ganascia and Gauvain Bourgne who are both Professor at Sorbonne University (Paris – France).
Contact: Jean-Gabriel.Ganascia@lip6.fr and Gauvain.Bourgne@lip6.fr
From more details click here.
Department of Computer Science at the University of Copenhagen offers one
fully funded PhD position on Data-driven Learning, Optimization and Control
of Pharmaceutical Production.
The PhD position concerns adaptive learning and control of complex
stochastic processes arising in pharmaceutical production to maximize the
production yield of such processes. This involves learning dynamics of the
stochastic process and devising adaptive algorithms to maximize the
objective, capable of achieving a near-optimal performance in the long run
and of adapting to process variations. To this end, the project uses and
advances methods from machine learning, (deep) reinforcement learning, and
adaptive control theory. The project will be carried out in close
collaboration with Novo Nordisk, a leading pharmaceutical company in
Denmark, thus offering a unique opportunity to work with real-world
production data.
The PhD position is planned to commence on March 1, 2021 or as soon as
possible thereafter. Detailed information regarding the position and the
application procedure can be found at:
https://employment.ku.dk/phd/?show=152947
Application Deadline: *December 15, 2020*